Telecom Analytics for Fraud Management and Consumer Protection: Customers and telecom companies alike are becoming increasingly concerned about telecom fraud and data breaches. As the use of mobile phones, data plans, and digital payments grows, so do the opportunities for fraud and cybercrime. Apart from financial losses, telecom fraud may adversely impair a company’s reputation and client confidence. To combat this, telecom analytics has evolved into a powerful tool for fraud detection and consumer protection.
In telecom analytics, advanced data analysis tools are utilised to identify patterns, trends, and anomalies in network activity and client behaviour. By analysing this data, telecom companies may improve customer happiness, secure their networks, maintain regulatory compliance, and detect and halt fraudulent actions. In this post, we’ll look at how telecom analytics may be used to help the telecom industry combat fraud and protect customers.
For telecom companies to maintain customer trust and loyalty, the use of telecom analytics for fraud management and consumer protection is essential. With the right tools and techniques, businesses can proactively detect and prevent fraud, safeguard customer data, and provide customised services that meet each customer’s unique needs and preferences.
Telecom Analytics for Fraud Management and Consumer Protection
Telecom analytics can be an effective tool for detecting and preventing fraud and protecting consumers in the telecommunications industry. Here are some ways that telecom analytics can be used for fraud management and consumer protection:
- Fraud Detection: Telecom analytics can be used to detect patterns and anomalies in call records, usage data, and other customer behavior that may indicate fraudulent activity. Machine learning algorithms can be trained on historical data to identify patterns of fraud and flag suspicious activity in real-time.
- Network Security: Telecom analytics can be used to identify security threats and vulnerabilities in the network infrastructure, such as hacking attempts or malware infections. This information can be used to proactively take measures to secure the network and protect customer data.
- Compliance Monitoring: Telecom analytics can be used to monitor compliance with regulatory requirements and industry standards, such as the Federal Communications Commission (FCC) rules and guidelines. This helps ensure that telecom companies are operating within legal and ethical boundaries and protecting consumer privacy.
- Customer Experience: Telecom analytics can be used to analyze customer feedback, complaints, and support requests to identify areas where improvements can be made to the customer experience. This helps companies deliver better service and support to their customers, leading to increased loyalty and satisfaction.
- Personalization: Telecom analytics can be used to analyze customer behavior and preferences to personalize offers and services to individual customers. This helps companies tailor their offerings to meet the unique needs and interests of their customers, leading to increased engagement and loyalty.
Various ways in which Telecom Analytics can be used for Fraud Detection
There are several approaches to use telecom analytics for detecting fraud in the telecoms sector. These are a few of the most popular techniques:
- Anomaly Detection: Anomaly detection is a machine learning method used to spot irregularities and trends in data that could be signs of fraud. Anomaly detection algorithms can spot unusual behaviour in real-time and notify fraud management teams by examining call logs, usage statistics, and other consumer behaviour.
- Network Traffic Analysis: Network traffic analysis is keeping an eye o ut for any strange patterns or behaviours that might point to malware infestations or hacking efforts. Companies can see possible risks and take precautions to secure their networks and safeguard client data by evaluating network data in real-time.
- Device Fingerprinting: To identify fraudulent activity, device fingerprinting analyses distinctive device attributes including device type, operating system, and browser version. Companies can stop future fraud attempts from those devices by recognizing the ones that have been previously used in fraudulent behavior.
- Behavioral analysis: The goal of behavioural analysis is to find trends in consumer behaviour that could point to fraudulent behaviour. Companies can identify changes in customer behaviour that might suggest fraud and take steps to avoid it by studying call records, use statistics, and other consumer activity.
- Link analysis is the process of locating connections between various accounts or gadgets that can point to fraudulent behaviour. Link analysis algorithms can find links between accounts or devices that might be implicated in fraud by examining call logs, usage information, and other consumer behaviour.
What is Fraud Detection for Telecommunication Industry?
Fraud detection for the telecommunications sector entails spotting and stopping potentially fraudulent activities inside the network, services, or billing systems. Several types of fraud fall under this category, including:
Subscription fraud is the practice of signing up for a mobile phone contract while posing as someone else or using a false identity.
Cloning fraud is when someone copies another person’s phone or SIM card so they may use the victim’s account to make calls, send messages, and access data.
Fraudulently forwarding calls from a genuine account to another phone number, which is then used to place or receive calls that are charged to the legal account, is known as call forwarding.
Roaming fraud is when someone uses a mobile handset abroad and pays expensive roaming fees without the legal account holder’s consent.
When someone subscribes to premium rate services without the genuine account holder’s knowledge or agreement, illegal charges are made to the account. This is known as premium rate service fraud.
In order to find trends and abnormalities in consumer behaviour and network activity that may point to fraudulent activities, the telecoms sector often uses advanced data analytics and machine learning techniques. Fraud management teams can take action to stop additional fraud by being alerted by fraud detection algorithms, which can identify suspect activity in real-time by monitoring call records, use statistics, and other consumer behaviour.
Protecting clients from financial loss and avoiding harm to the telecoms company’s reputation are the two main objectives of fraud detection in the sector of telecommunications.
What are the ways of Fraud Detection?
There are several ways of fraud detection, including:
- Rule-based detection: In this method, criteria or rules are established based on past data to spot possibly fraudulent conduct. For instance, it may be a sign of fraud if a customer’s use quickly rises over a specified level. The action would then be flagged by the system for additional examination.
- Anomaly detection uses machine learning techniques to find unexpected patterns in data that might be signs of fraud. For instance, it can be a sign of fraud if a consumer suddenly starts using their phone at odd times or places. The action would then be flagged by the system for additional examination.
- Link analysis: This method looks for patterns of fraudulent conduct by examining links between various accounts, gadgets, or transactions. For instance, it can be a sign of fraud if many accounts are using the same credit card or phone number. The action would then be flagged by the system for additional examination.
- Fraud detection based on machine learning: With this method, machine learning algorithms are used to evaluate huge amounts of data in real-time and spot possibly fraudulent activities. The algorithms are capable of learning from prior data and adapting to new fraud patterns, which makes them more efficient over time.
- Social network analysis: In this method, relationships between people are examined in order to spot probable fraud rings or networks. For instance, it can point to a fraud network if several people are linked to one another through several accounts or transactions. The action would then be flagged by the system for additional examination.
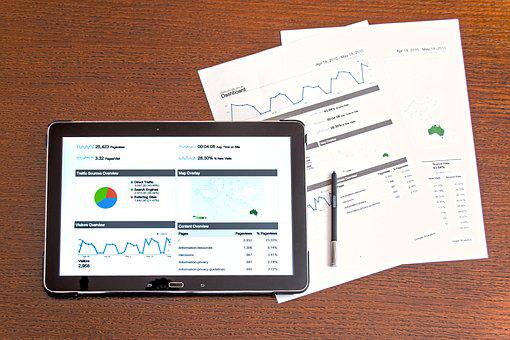
While telecom analytics may be an effective tool for managing fraud and protecting consumers, there are also a number of difficulties and restrictions that come with using it.
The following are some of the primary issues and restrictions:
Data quality: Ensuring the quality of the data being analysed is one of the main issues when employing telecom analytics for fraud management and consumer protection. False positives or lost opportunities for fraud detection might result from incomplete, incorrect, or inconsistent data.
Complexity: Because telecom networks and services are dynamic and complicated, it is difficult to create fraud detection algorithms that can keep up with constantly changing threats.
Data privacy: As telecom analytics makes use of sensitive consumer data, businesses must make sure they are adhering to all relevant data privacy laws. If you don’t, you risk legal trouble and harm to your reputation.
Cost: Setting up a powerful telecom analytics system may be pricey, and smaller businesses might not have the funds to invest in the required equipment and knowledge.
False positives: Successful fraud detection algorithms must strike a compromise between the requirement to spot potentially fraudulent behaviour and the danger of producing false positives, which can result in unhappy customers and higher operating expenses.
Threats that are continuously changing: To avoid being caught, fraudsters are continually coming up with new strategies and tools, making it difficult for fraud management teams to keep on top of new dangers.
While telecom analytics may be a useful tool for managing fraud and protecting consumers, businesses must be aware of these difficulties and limits and come up with solutions. This may entail funding projects to improve data quality, guaranteeing adherence to data privacy laws, implementing a risk-based strategy for fraud detection, and continuously monitoring and improving fraud detection algorithms to handle new threats.
In conclusion, fraud and data breaches pose a growing danger to the telecommunications sector and can harm businesses and their clients financially and reputationally. Yet, businesses may proactively identify and stop fraudulent behaviour, safeguard their networks, guarantee regulatory compliance, and enhance the customer experience by adopting telecom analytics.
Advanced data analysis methods are used in telecom analytics to find patterns and irregularities in network activity and consumer behaviour. Machine learning algorithms enable businesses to examine massive amounts of data in real-time and come to wise conclusions that safeguard both their consumers and their operations.
Businesses may increase client trust and loyalty, safeguard the reputation of their brands, and detect and prevent fraud. Telecom analytics may also assist businesses in tailoring their offerings to each client’s particular requirements and preferences, which will enhance client engagement and loyalty.
The advantages of adopting telecom analytics for fraud control and consumer protection exceed the dangers by a wide margin, notwithstanding some difficulties and restrictions related to data privacy and regulatory compliance. Telecom analytics will become more crucial as the telecoms sector develops in safeguarding customers and companies from fraud and data breaches.