Data science field popularity and demand for data scientists has been growing rapidly from past many years, since the importance of data has been recognized by organisations. Many professionals choosing Data Science as their career growth path. But, what if you want to earn extra money from your data science skills or what are the ways to make money from your data science knowledge?
For organisations, companies and businesses of all sizes, data science has become a crucial part of the decision-making process. Data scientists have been paid well as a result of their high demand. Apart from this, Data scientists can monetize their skills, knowledge and earn more by solving business problems and helping others.
In this article, we will explore top 10 additional data science revenue streams, side hustles or ways to make money, which helps you in earning extra nuggets and pizzas for your appetite and financial goals.
Some of them include: Providing consulting services, creating tailored solutions, building data visualizations, working as a data engineer, developing products for solving business problems, conducting data analysis, developing predictive models, and teaching data science fundamentals, topics and principles to students or learners.
By pursuing one or more of the following, data scientists might find fresh and exciting ways to use their skills, share their knowledge, enhance their career and earn more money. Let’s dig in!
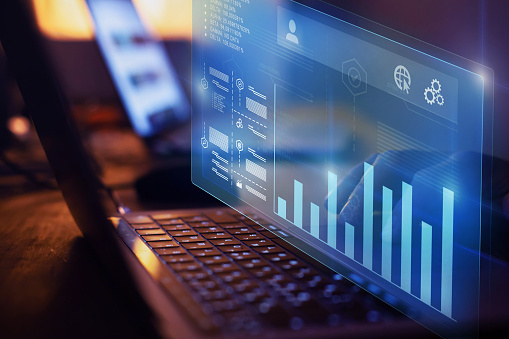
Table of Contents
- Top 10 Ways to Make Money from Data Science
- 1. Data Science Consulting
- 2. Developing Custom Data Science Solutions
- 3. Data Visualization
- 4. Machine Learning as a Service (MLaaS)
- 5. Data Engineering
- 6. Developing data products
- 7. Provide Data Analysis Solutions or Services
- 8. Building AI Models, AI Apps or AI Solutions
- 9. Developing Machine Learning (ML) Predictive Models
- 10. Provide Data Science Training
- Conclusion
Top 10 Ways to Make Money from Data Science
Here are the top 10 effective ways to make money or earn extra money from data science:
1. Data Science Consulting
Data science consulting is the process of providing your knowledge to companies or businesses, in order to assist them in improving their decision-making processes and solving challenging challenges using data,
As a data science consultant, you can collaborate directly with customers to discover data-related difficulties and design customised solutions to fit their unique requirements.
But for this role, you should have a solid foundation in data science, including experience in data analysis, machine learning, data visualisation, and how to solve a business problem and providing them efficient solution. You should capable in dealing effectively with clients with good communication skills and problem-solving abilities.
You can work as a data science consultant with a variety of customers, including small startups, major enterprises, and government organisations. You can provide your services on a project basis or as a continuing consultant.
Data science consulting offers the opportunity to work on a range of fascinating projects, choose your own schedule, and make a good salary. It does, however, need a high level of discipline, since you will need to manage your own firm and locate new clients on a regular basis.
2. Developing Custom Data Science Solutions
Creating custom data science solutions entails creating specialised software and apps to assist organisations in solving specific challenges with data. You will collaborate with customers to understand their needs and build a solution that uses data to help them make better decisions and achieve their objectives as a bespoke data science solution developer.
A solid experience in data science, including competence in machine learning, data analysis, and data visualisation, is required to become a bespoke data science solution developer. You should also be familiar with programming languages such as Python, R, and SQL, as well as data-related tools and technologies such as Hadoop, Spark, and TensorFlow.
Custom data science solution development is often done on a project basis, with clients ranging from tiny startups to mid-sized businesses to huge enterprises. Fraud detection systems, recommendation engines, and predictive analytics tools are popular examples of specialised data science solutions.
The advantages of generating bespoke data science solutions include the opportunity to work on fascinating and demanding projects, the capacity to create new solutions that solve real-world issues, and the possibility of earning a high salary.
It does, however, need excellent project management abilities as well as the ability to successfully collaborate with customers in order to understand their needs and build solutions that fit their specifications.
3. Data Visualization
The process of developing useful and appealing visual representations of data to help people better understand and grasp complicated information is known as data visualisation.
As a data visualisation specialist, you will utilise a number of tools and techniques to generate visually appealing charts, graphs, and other visualisations that aid in data interpretation. A solid foundation in data analysis and visualisation, as well as familiarity with data visualization technologies such as Tableau, Power BI, or D3.js, are required to become a data visualisation professional. You will also require an eye for design as well as exceptional communication skills to communicate complicated facts in an understandable manner.
You may work with a wide range of customers as a data visualisation specialist, including small enterprises, major organisations, and government agencies. Dashboards, infographics, and interactive visualisations are some forms of data visualisations.
The capacity to produce interesting and informative visualisations that help people better grasp difficult information, the opportunity to work on a range of projects in different sectors, and the possibility for a high salary are all advantages of working in data visualization. However, it also necessitates a keen attention to detail, as well as the ability to operate under pressure and successfully interact with clients.
4. Machine Learning as a Service (MLaaS)
Machine learning as a service (MLaaS) is a cloud-based service that provides machine learning capabilities to clients. You will give clients with access to pre-built machine learning models and tools that they may use to construct their own unique solutions as an MLaaS provider.
You’ll need a solid expertise in machine learning as well as knowledge with cloud computing platforms like Amazon Web Services (AWS), Microsoft Azure, or Google Cloud Platform (GCP) to provide MLaaS.
You will also require a great grasp of your clients’ demands, as well as excellent project management abilities, to guarantee that you offer high-quality services on time and under budget.
You may deal with a wide range of clients as an MLaaS provider, including startups, mid-sized businesses, and major enterprises. MLaaS applications that are commonly used include fraud detection, consumer segmentation, and predictive maintenance.
The advantages of providing MLaaS include the ability to provide powerful machine learning capabilities to clients without requiring them to have extensive expertise in this area, the potential for recurring revenue through subscription-based pricing models, and the ability to scale your services rapidly as your client base grows.
However, it also necessitates a substantial investment in cloud infrastructure as well as constant maintenance and support to guarantee that your services stay current and reliable.
5. Data Engineering
Data engineering is a branch of data management that comprises the design, construction, testing, and upkeep of the infrastructure and architecture required for collecting, storing, processing, and analysing huge volumes of data. Data engineering is an important part of modern data-driven organisations because it ensures that data is correctly acquired, cleansed, and turned into a format that data analysts and data scientists can use.
Databases, data warehouses, data lakes, data integration solutions, ETL (extract, transform, load) and ELT tools, and big data technologies such as Hadoop and Spark are all used by data engineers. They are also in charge of guaranteeing the data infrastructure’s dependability, scalability, and security, as well as building and managing data pipelines to transmit data between systems and applications.
Data engineering is essential for organisations to make data-driven choices, increase operational efficiency, and develop new business prospects. Data engineering will remain an essential and fast expanding discipline as data quantities continue to rise and organisations increasingly rely on data to drive their operations.
6. Developing data products
The process of producing software applications, tools, and services that use data and machine learning models to deliver important insights or conduct automated activities is referred to as developing data products. These solutions are intended to address certain business problems, automate processes, or give actionable information for decision making.
Data product development involves several steps, including:
- Identifying the business problem or opportunity: This involves understanding the specific business problem or opportunity that the data product aims to solve or address.
- Data collection and cleaning: This involves gathering and preparing the data necessary for building the model or tool. The data must be cleaned and prepared to ensure that it is accurate and relevant.
- Model building and training: This involves building machine learning models or developing algorithms that can process the data and provide valuable insights. The models are trained using historical data to ensure they provide accurate results.
- Deployment: Once the model is built and trained, it needs to be deployed in a production environment where it can be accessed by users.
- Monitoring and maintenance: Once the product is deployed, it must be monitored and maintained to ensure it remains accurate and reliable.
7. Provide Data Analysis Solutions or Services
The process of reviewing, cleaning, manipulating, and modelling data in order to identify usable information, make conclusions, and help decision-making. It entails using statistical and computational approaches on vast amounts of data to discover insights, patterns, and trends. A range of approaches, such as descriptive statistics, inferential statistics, data mining, machine learning, deep learning and data visualisation, can be used to analyse data.
Data analysis process starts with collecting essential data. Data collectiion entails gathering information from numerous sources such as surveys, databases, third party sources and social media.
Data cleaning is the process of removing or repairing mistakes, inconsistencies, or missing information from data. To aid data analysis, data collection and data preparation steps helps in transforming raw data into a more organised format, such as a database or spreadsheet.
8. Building AI Models, AI Apps or AI Solutions
Creating AI models and apps using AI technology consist of developing algorithms or software programs that can learn from data and make predictions, forecasting or judgement based on it. This procedure consists of multiple phases, including:
- Data collection and preparation: Gathering and organizing data that will be used to train the AI model. This includes cleaning and preprocessing the data to ensure it is suitable for use.
- Algorithm selection and customization: Choosing an appropriate machine learning algorithm based on the type of problem to be solved, and customizing it to suit the specific requirements of the problem.
- Model training: Feeding the algorithm with the preprocessed data and tuning it to optimize the model’s performance on the given problem.
- Evaluation and validation: Testing the model’s performance using a separate dataset and measuring its accuracy and reliability.
- Deployment and monitoring: Integrating the model into a production environment and monitoring its performance in real-time.
These AI apps or solutions help companies or individuals to predict something which can be helpful to them and assist them in deriving decisions.
9. Developing Machine Learning (ML) Predictive Models
Predictive modelling is a sort of data modelling that includes utilising previous data to anticipate future occurrences or outcomes. Predictive modelling is a subset of machine learning that forecasts trends and behaviors, identifies patterns, and informs decision-making processes.
The process of developing predictive models includes the following steps:
- Define the issue: Determine the business problem or question that will be addressed using predictive modelling. This might include estimating sales, anticipating customer attrition, or identifying fraud.
- Data collection entails gathering the required information from numerous sources, such as databases, sensors, or client feedback.
- Data preparation entails cleaning and preprocessing data to assure accuracy, relevance, and consistency. This might include deleting missing numbers, outliers, and duplicates, as well as converting the data to a different format.
- Identify and pick the most relevant features or variables to include in the model using feature engineering. This might include feature selection, extraction, or transformation.
- Model selection: Based on the issue to be addressed and the data available, pick the best machine learning method. This may entail comparing and assessing several methods to get the greatest match.
- Model training: Build the prediction model by training the chosen algorithm on historical data. This may entail dividing the data into training and validation sets and optimising the model’s performance with techniques such as cross-validation and regularisation.
- Model evaluation: Evaluate the prediction model’s performance and accuracy on a distinct dataset. This might include comparing the model’s predictions to actual outcomes or utilising measures like precision, recall, and accuracy.
- Deployment: Integrate the predictive model into a production setting and continuously monitor its performance. It may be necessary to automate the model’s predictions and incorporate them into business processes or decision-making systems.
10. Provide Data Science Training
Data science is an interdisciplinary subject that uses statistics, mathematics, computer science, and domain expertise to extract insights and information from data. If you want to work in data science, there are numerous steps you may take to obtain the essential skills and knowledge:
Understand the fundamentals: Begin by understanding the fundamentals of statistics, programming, and data analysis. This can be accomplished through the use of online courses, tutorials, or textbooks. Coursera, edX, Khan Academy, and DataCamp are other prominent options.
Practise with actual data: Once you’ve mastered the foundations, go on to real-world datasets. Kaggle is a fantastic tool for finding datasets and competing in tournaments.
Choose a specialisation: Machine learning, data engineering, big data, and business analytics are just a few of the areas covered by data science. Select a specialisation depending on your interests and professional objectives.
Develop your programming skills: Data scientists are expected to be fluent in programming languages like Python, R, and SQL. Build data pipelines to combine data from numerous sources and practise coding on actual datasets.
Create a portfolio of projects to demonstrate your abilities and knowledge. Showcase your creations on platforms like as GitHub, LinkedIn, or Kaggle.
Connect with other data scientists in the business through networking. To become active in the data science community, attend meetings, join online communities, and participate in forums.
Considering about formal education: Study data science or a similar topic to obtain a thorough knowledge of the concepts and principles that underpin data science. Master of Data Science, Master of Business Analytics, and Master of Science in Computer Science are other common programmes.
👉 You may also like: Key Highlights from the Gartner Data Analytics Summit
Conclusion
In conclusion, data science has become a highly valuable skill in today’s digital economy. The ability to analyze, interpret and draw insights from data has made it possible to leverage the vast amounts of information available to businesses and organizations. As a result, data scientists are in high demand, and there are many ways to make money from data science.
To succeed in any of these areas, it is crucial to develop a strong foundation in data science skills such as programming, statistics, and machine learning. You must also continually improve your skills and stay up to date with the latest trends and technologies.
The opportunities for making money from data science are vast and varied, and the field is continually evolving. With hard work, dedication, and a commitment to learning, anyone can turn their passion for data science into a profitable career and start earning extra income.
[…] Developing these skills will help you become ready for a range of data entry jobs, including freelance work and roles as an administrative assistant. This article covers both the technical skills and soft skills, necessary for success in these professions as it analyses the key competencies needed to thrive in the data entry industry. […]