The raw and rich types of data or big data that companies generate, contain valuable insights, market trends and patterns. Data analytics is the best way to unlock them. Data Analytics and Data Analysis within can help organizations to refine marketing strategies, enhance operational efficiency, and mitigate potential risks. By effectively utilizing data analytics strategies and techniques, organizations can uncover right patterns, predict latest markets trends, and make data-driven decisions.
In this article, we will explore the advantages and disadvantages of data analytics (data analysis). What is the impact of data analysis on decision-making, efficiency, and business growth, while addressing its limitations.
Table of Contents
Data Analytics Vs. Data Analysis
Many people especially students and freshers, often get confused between these two terms: Data Analytics and Data Analysis and sometimes use them interchangeably. Although, both belongs to the same subject but have different characteristics and process.
Data analysis is a critical component of the broader and complete data analytics process. Data analysis refers to the process of examining, interpreting, and extracting insights from data, whereas data analytics encompasses the entire lifecycle of processing data, including data collection, merging, processing, preparing data, analysing data and application of analytical models (Machine learning algorithms and AI).
Our focus here is specifically on data analysis advantages and disadvantages, rather than the complete data analytics framework.
Data Analysis Advantages:
Let’s look at the five advantages of using data analysis:
1. Customize the customer experience
Companies collect customer data through many different channels, including physical shopping, e-commerce, and social media. Take a clothing business that has a physical and online presence. The business can analyze its sales data and data from its social media pages and create targeted advertising campaigns to promote its online sales for the types of products that customers are interested in.
Organizations can perform behavioral analysis on customer data to further enhance the customer experience. For example, businesses can perform predictive modeling on e-commerce transaction data to determine which products to recommend at checkout to increase sales.
2. Communicate business decisions
Businesses can use data analysis to guide business decisions and reduce financial losses. A forecast analysis can suggest possible actions in response to business changes, and a written summary can show how the business should react to those changes.
For example: A company may create changes in price or product offerings to determine how those changes will affect customer demand. Changes in product offerings can be A/B tested to validate the ideas generated by this model.
After collecting market data on modified products, companies can use data analysis tools to determine the success of the changes. Then, after visualizing the results decision-makers can decide, whether to implement new findings or changes in the next marketing campaigns.
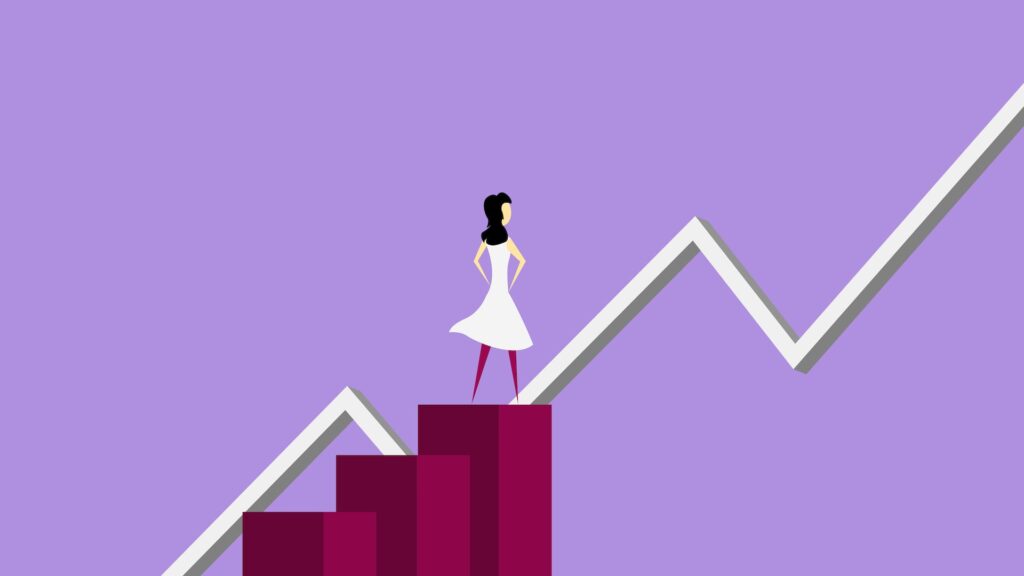
3. Streamline operations
Organizations can improve their performance through data analysis. For example: Collecting and analyzing supply chain data can show where production delays or bottlenecks are occurring and help predict where problems may arise.
By considering these factors, company can decide if the demand forecast indicates that one supplier will not be able to handle the volume required for the holiday season, the company may add or replace the supplier to avoid production delays by keeping enough inventory stock.
In addition, many businesses, especially in retail, often struggle to maintaining and improve their inventory levels. Data analysis can help identify the best sources for an entire company’s products inventory based on factors such as future demand, seasonality, holidays, and global trends.
4. Reduce risk and manage setbacks
Risk is everywhere in business. For example, a retail chain may use predictive modeling – a statistical tool (part of machine learning) that can predict future events or activities – to determine which stores are most at risk of the week. theft. The company can use this data to determine the level of security in the store, or even if it will remove itself from certain areas.
Investors can also use data analysis to reduce losses after a pullback. If a business is overstocking a product, it can use data analysis to determine the best price for tracking to reduce inventory. A company can create a statistical model to provide immediate advice on how to solve common problems.
5. Improved security
All businesses face data security risks. Organizations can use data analysis to determine the cause of past data breaches by organizing and using relevant data views. For example, IT can use data analysis tools to analyze, organize, and visualize its audit logs to determine the timing and origin of an attack. This information can help IT find vulnerabilities and fix them.
IT departments can also use statistics to prevent future attacks. Attacks often involve malicious access behavior, especially payload-based attacks such as denial-of-service (DDoS) attacks. Organizations can configure these models to be continuous, with monitoring and evaluation systems to identify and report anomalies so that security professionals can take immediate action.
Data Analysis Disadvantages:
Let’s look at some of the disadvantages of using data analysis:
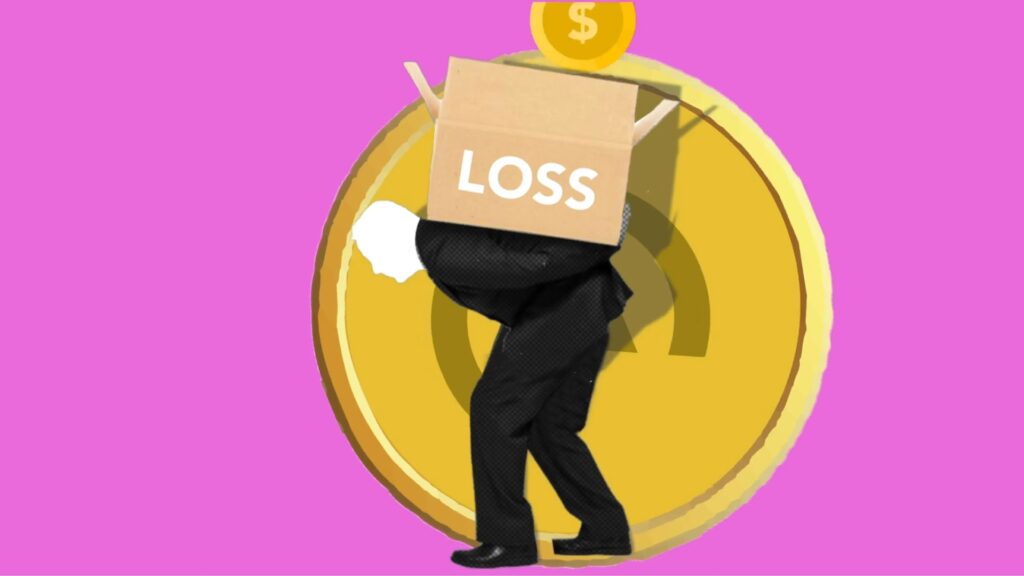
1. Lack of coordination
Forecasting results may provide wrong predictions for product demand or poor investment decisions or may lead to incorrect diagnoses, because of lack of coordination between different groups or departments within an organisation.
Data analysis can be done by members of the working group and the analysis can be shared with the administrative staff. However, the information these groups produce separately without proper coordination is not valuable or has much impact on organizational metrics.
This may be due to the way work is done in “silos” and each group uses only their current system and data, disconnected from other departments of the organisation. While the research team will focus on answering the key business questions, they are often limited by the data available to them.
Every team, whether it is data collection team, database team, data analysis team or data science team, must ensure that the right data is used for predictions and insights, after properly data collection and coordination with all the concerned teams. And, the final results should be effectively communicated to the right employees.
Without the right coordination and collaboration, businesses will risk making decisions based on incomplete or fragmented information.
2. No guarantees and patience
Data collection solutions are not difficult to implement, but they are expensive and the return on investment is not fast. In particular, if existing data is not available, creating a system and process to start collecting data can take time.
By nature, research models improve accuracy over time and require dedication to implement the solution. Since consumers don’t see immediate results, they sometimes lose interest, which leads to lack of confidence and failure.
When a company decides to implement a data analysis system, it is important to use a feedback system in order to understand what is working and what is not. It will help in identifying improvements which are needed to fix it.
Without this structured and closed process, the management is forced to take tough decisions and decide that the research is ineffective or not useful. Which may result in abandoning the entire exercise or dissolving the respective teams.
3. Low data quality
One of the main limitations of data analysis is the bad or dirty data and lack of access to quality data. One of the most impediments of information investigation is the need of get to to quality information. Companies may as of now have get to to a part of information, but the address is, do they have the correct information they require?
A top-down approach is required where the trade questions that ought to be replied must to begin with be known and the information required to reply those questions can be decided. In a few cases, the information may be collected for authentic purposes and may not be sufficient to reply the questions we are inquiring nowadays.
At other times, even though we have the proper measurements to gather information from, the quality of information collection can be destitute. There may be circumstances where adequate information isn’t accessible or lost to perform a appropriate investigation.As they say, trash goes out.
In case the quality of the information is terrible, the choices made to utilize the information will too be terrible. Hence, steps must be taken to make strides in the quality of information, so that it can be utilized viably in an organization.
4. Privacy Concern
Sometimes the collection of data can compromise the privacy of customers because their information such as purchases, online transactions, and subscriptions are available to companies that use their services. Some companies may exchange these data sets with other companies for mutual benefit. Some of the data collected may be used against an individual, a country, or a country.
Organizations should be careful about the type of data they collect and share from customers, it will ensure data security and privacy. Only the data required for analysis should be captured and shared. When there is sensitive data, it should be annotated so that sensitive data is protected. Data breaches can cause customers to lose trust in organizations, which can negatively impact the organization.
5. Confusion and uncertainty
Some research tools developed by companies are like black box models. It is not clear what is inside the black box or what is the actual concept (logic) of the system that learns the data and creates a final model. For example, a type of neural network algorithm that learns from data based on different situations to decide, who should receive money and who should refuse!
Using such models can be enticing, but if the logic behind making decisions is not clearly understood by everyone in the business, it may lead to misinterpretations, poor strategic choices and poor results.
Further Advantages and Disadvantages of Data Analysis
By understanding the various benefits and challenges associated with data analysis, organizations can make informed decisions and devise effective strategies to harness the power of data for effective decision making. Let’s uncover data analysis more advantages and disadvantages to understand it better –
Data Analysis Advantages | Data Analysis Disadvantages |
---|---|
1. Informed Decision Making: Data analysis helps organizations to make informed decisions based on factual insights and trends derived from data. | 1. Data Quality Issues: Poor data quality may lead to inaccurate analysis and results in business losses. |
2. Identifying Patterns and Trends: Data analysis helps identify patterns, trends, and relationships within datasets, providing valuable insights. | 2. Data Complexity: Analyzing large and complex datasets (big data) can be challenging and time-consuming, requiring specialized skills and tools. |
3. Improved Efficiency: By analyzing data, organizations can identify inefficiencies, streamline processes, and improve overall operational efficiency. | 3. Data Privacy and Security: Handling sensitive information raises concerns about data privacy and security. Protecting data from unauthorized access and breaches is crucial for every organization. |
4. Better Customer Understanding: Data analysis helps organizations gain a deeper understanding of their customers needs, future demands, preferences, and behaviors. | 4. Biases and Misinterpretation: Data analysis is subject to biases and misinterpretation. Presence of bias in data may lead to flawed conclusions and incorrect decisions. |
5. Competitive Advantage: Utilizing data analysis provides a competitive edge by uncovering insights that can drive innovation, marketing strategies, and business growth. | 5. Cost and Resource Intensive: Implementing and maintaining data analysis team with right capabilities can be costly, requiring investments in tools, technologies, and skilled personnel. |
Conclusion:
In today’s data-driven world, predicting future demand and customer behavior is crucial for any business success. Right implementation of data analysis processes help organizations to identify future trends, improve production efficiency, offer effective treatment plans, launch effective campaigns, make better data-driven decisions and gain a competitive edge over others.
Apart from offering several advantages, improper implementation of data analysis processes and techniques results in certain challenges or disadvantages, such as data quality issues, privacy concerns, biases, and resource-intensive implementation
To maximize the benefits of data analysis, businesses must ensure data accuracy, invest in skilled professionals, and implement strong security measures. By balancing data analysis advantages and disadvantages, organizations can take right step forward for better efficiency and greater business results.
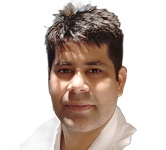
Kunal Aneja is the Head of DataBonker.com, leading the platform’s vision to provide informational and educational resources on Education, Career, Jobs, Data Analytics, Cloud Computing, AI, and Technology. With a deep passion for knowledge-sharing and innovation, Kunal is dedicated to delivering insightful, research-driven content that helps individuals navigate their professional and academic journeys. Under his leadership, DataBonker continues to evolve as a trusted hub for learning resources, career guidance, and tech insights.
📩 **Stay Ahead with DataBonker!**
Join our newsletter and get the latest insights on "Education, Admissions Guidance, Career Growth, Latest Job Trends, and Technology Updates** – straight to your inbox! 🚀